When 250,000 participants aren't better than 10
Plus overconfident digital brains in the Inaugural Edition.
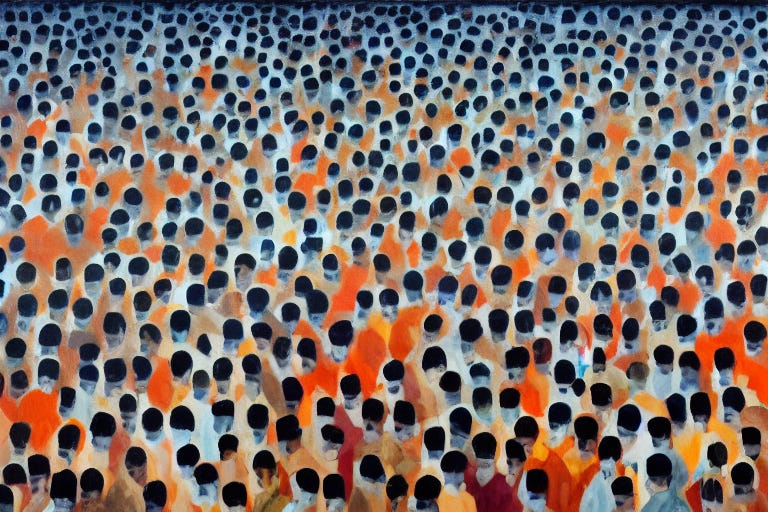
Hello, world!
This is the first issue of the newsletter, and… well, it's likely been a bit since you subscribed. In case you forgot: I'm Lawton Pybus.
Thank you for being an “early adopter!” It means a lot. Going forward, I’ll be sending these on every third Sunday of the month.
A thought on… beer money and sampling bias
A lot of my day job involves complex study designs, reaching impossible-to-find participants, and doing it all at the scale of massive sample sizes. So I end up thinking a lot about how hard it is to make sure we’re reaching the right people for the best data.
Beyond the standard advice for “how many participants do we need?”, the composition of that sample becomes extremely important. From Nature this month:
A survey of 250,000 respondents can produce an estimate of the population mean that is no more accurate than an estimate from a simple random sample of size 10. Our central message is that data quality matters more than data quantity,1 and that compensating the former with the latter is a mathematically provable losing proposition.
How does something like this happen? In short, no matter the sample size, GIGO applies. The authors continue: “When biased samples are large, they are doubly misleading: they produce confidence intervals with incorrect centres and substantially underestimated widths.”
There was a great example of this a few months back when a viral TikTok video about easy ways to make some beer money ended up throwing off dozens of ongoing academic studies conducted on the popular participant sourcing platform, Prolific. The creator’s predominantly female audience flooded the panel, skewing demographics in studies that didn’t explicitly control for it. (Meanwhile, in our field, filling studies using any non-essential demographic data is becoming an increasingly controversial practice.)
We don’t necessarily need representative samples, but in my experience, researchers don’t think critically enough about sampling bias beyond asking a few standard behavioral screening questions, or (more rarely) including some carefully-worded attention checks. In fact, there are many subtle ways you can construct your study to invite bias, whether in the questions and tasks themselves or in how and where you’re recruiting.
I propose an exercise to bring one facet of this closer to home: sign up as a study panelist2—not for the incentives, but to understand the participant experience. Dive into some of the communities where these folks hang out and try to understand their motivations and priorities. What makes a study satisfying or disappointing? Why would they spend 25 minutes doing tasks and answering questions on your site at all?
You’ll naturally start to think about and design your studies differently. It’ll help you understand on a visceral level why, for example, that niche B2B survey isn’t filling as fast as your team would like. And it’ll make you a better researcher.
What I’m up to: Scaling career advice
I spent a lot of this year taking on requests for “coffee chats” from juniors messaging me on LinkedIn, or more formal mentoring calls via ADPList.3 When I found myself repeating similar advice over and over, I decided to write it down.
The hope is that reading a 5-minute article will help a lot more people than booking 15 minutes with me. Here’s the latest:
Becoming a UX researcher out of grad school? Your advantages and disadvantages
Don’t assume that those extra letters after your name are an unmitigated advantage. In fact, grads carry some baggage. I break down what hiring managers see as the pro’s and con’s, and give suggestions for how to position yourself.
Thinking of going into UX research? 3 questions to help you decide
If your goal is to become a UX researcher, don’t make the hiring manager wonder if you’d actually prefer a design role. If you’re not sure if you want to specialize, I make the case why you should, and offer some advice to help you figure that out.
ICYMI: Should you have a UX research portfolio?
They may help you land your first role, but how many folks keep them up? I surveyed the field and conducted a job analysis to find out, and include some recommendations.
And more to come! I welcome your feedback, and encourage you to share if you think someone may find them useful.
The Distractor: A new source-monitoring error?
(Right, so this will be a section for neat stuff tangential to the topic of UX research.)
From PNAS: People mistake the Internet’s knowledge for their own
Those who use Google predict that they will know more in the future without the help of the internet, an erroneous belief that both indicates misattribution of prior knowledge and highlights a practically important consequence of this misattribution: overconfidence when the internet is no longer available.
I’ll file this under evidence for the theory that 21st century minds—whose boundaries we believe already to encompass the whole body, and at times even blur into other people—now have a digital element as well.
Tell me what you think.
So now you get the general idea of this newsletter, but nothing’s set in stone. Let me know what you like and what you don’t. There aren’t that many people on the list, so feel free to just hit reply. And if you do like where this is headed, please share it with someone else.
Here’s something specific I’m chewing on: is it ever justifiable to ignore behavior and only listen to what participants say in a user research study?
Wishing you all the best as we close out 2021 and look ahead to 2022.
Until then,
Lawton
Drill deeper
Does your team have more research requests than it can handle? Are there gaps in the team’s experience and skill set? Drill Bit Labs offers consulting and UX research services for ambitious teams. Hit reply and let’s elevate your practice together.
Emphasis mine, here and in later quoted text.
I’m suggesting Prolific here since a lot of those studies are basic academic ones. But generally, you know, be cool, and don’t intentionally contaminate fellow researchers’ data with your domain expertise, etc.
FWIW, I’m still taking on requests via ADPList, and I’m enjoying the conversations a lot. 2022 is looking busier though, so I’m not sure how much longer I can keep it up. So if you or someone you know may be interested, book some time now—it’s free.